hrnet相关的两篇文章
CVPR2019 Deep High-Resolution Representation Learning for Human Pose Estimation
High-Resolution Representations for Labeling Pixels and Regions (https://arxiv.org/pdf/1904.04514.pdf)
提出了一种新的架构,即高分辨率网络(HRNet),它能够在整个过程中维护高分辨率的表示。我们从高分辨率子网作为第一阶段始,逐步增加高分辨率到低分辨率的子网(gradually add high-to-low resolution subnetworks),形成更多的阶段,并将多分辨率子网并行连接。在整个过程中,我们通过在并行的多分辨率子网络上反复交换信息来进行多尺度的重复融合,使得每一个高分辨率到低分辨率的表征都从其他并行表示中反复接收信息,从而得到丰富的高分辨率表征.
网络示意图如下:
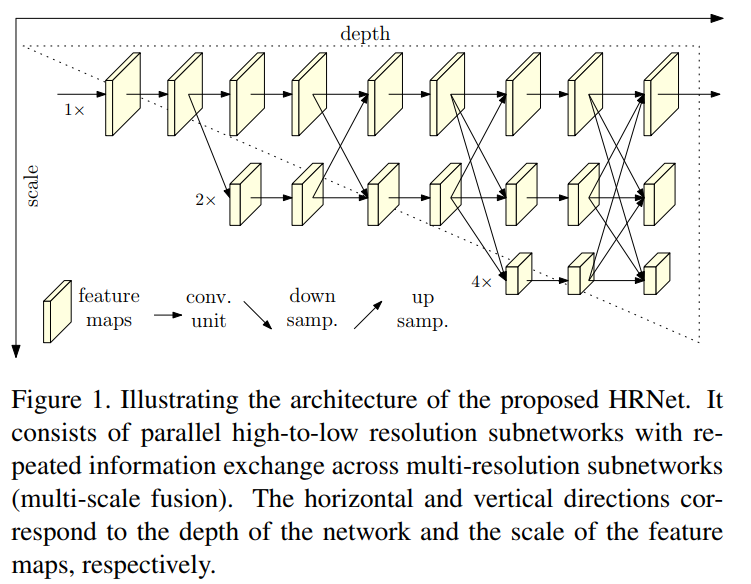
本文的贡献点:
1)我们的方法是,平行地连接从高到底分辨率的子网络,与目前绝大多数串联连接的网络不同。因此,我们的网络可以维持高分辨率特征,而不是通过一个从低到高的操作来恢复出一个高分辨率特征。 因此,预测的热图(heatmap)在空间上可能更精确。
2) 大多数现有的融合方案都是聚合低层(low-level)和高层(high-level)的表示( representations)。而本文提出的网络,使用重复的多尺度融合, 利用相同深度和相似级别的低分辨率表示来提高高分辨率表示。
(i) Our approach connects high-to-low resolution subnetworks in parallel rather than in series as done in most existing solutions. Thus, our approach is able to maintain the high resolution instead of recovering the resolution through a low-to-high process, and accordingly the predicted heatmap is potentially spatially more precise.
(ii)Most existing fusion schemes aggregate low-level and highlevel representations. Instead, we perform repeated multiscale fusions to boost the high-resolution representations with the help of the low-resolution representations of the same depth and similar level, and vice versa, resulting in that high-resolution representations are also rich for pose estimation. Consequently, our predicted heatmap is potentially more accurate.
网络结构如下图所示。
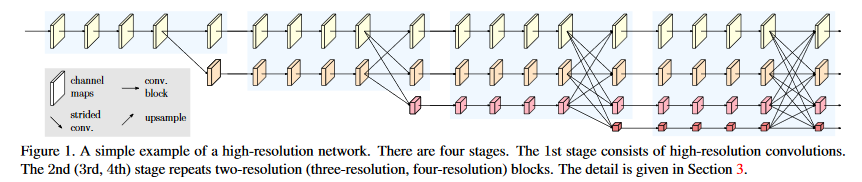
高分辨率与低分辨率的融合方式如下:
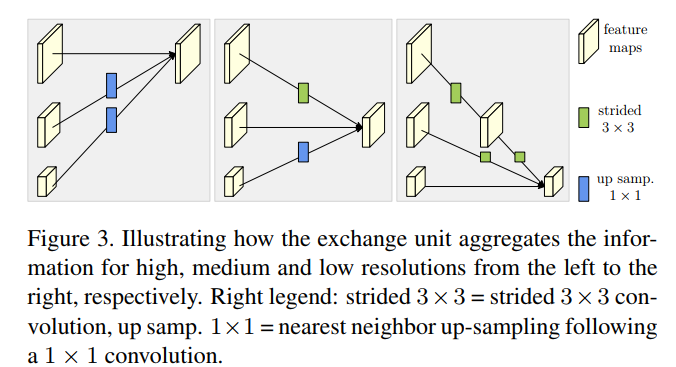
高分辨需要先用一个或者若干个连续的stride=2的3x3卷积(2个连续的stride=2的3x3卷积为 4倍的 downsampling)降低到与低分辨率相同,然后使用element wise sum,对不同分辨率进行求和。
低分辨率要先用一个上采样(Upsample,使用最近邻插值,使用2倍或者4倍的上采样率)的方式提升到与高分辨相同,然后使用1x1卷积使得通道数与高分辨一致,然后再进行sum操作。
最终不同分辨率的输出融合方式如下图:
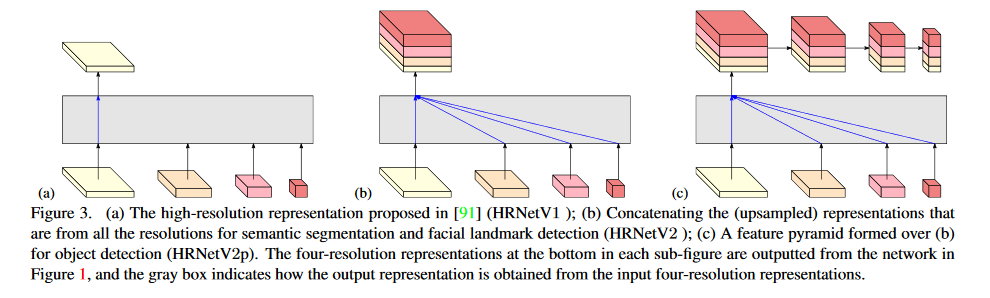
最后在coco 人形关键点检测的结果如下:
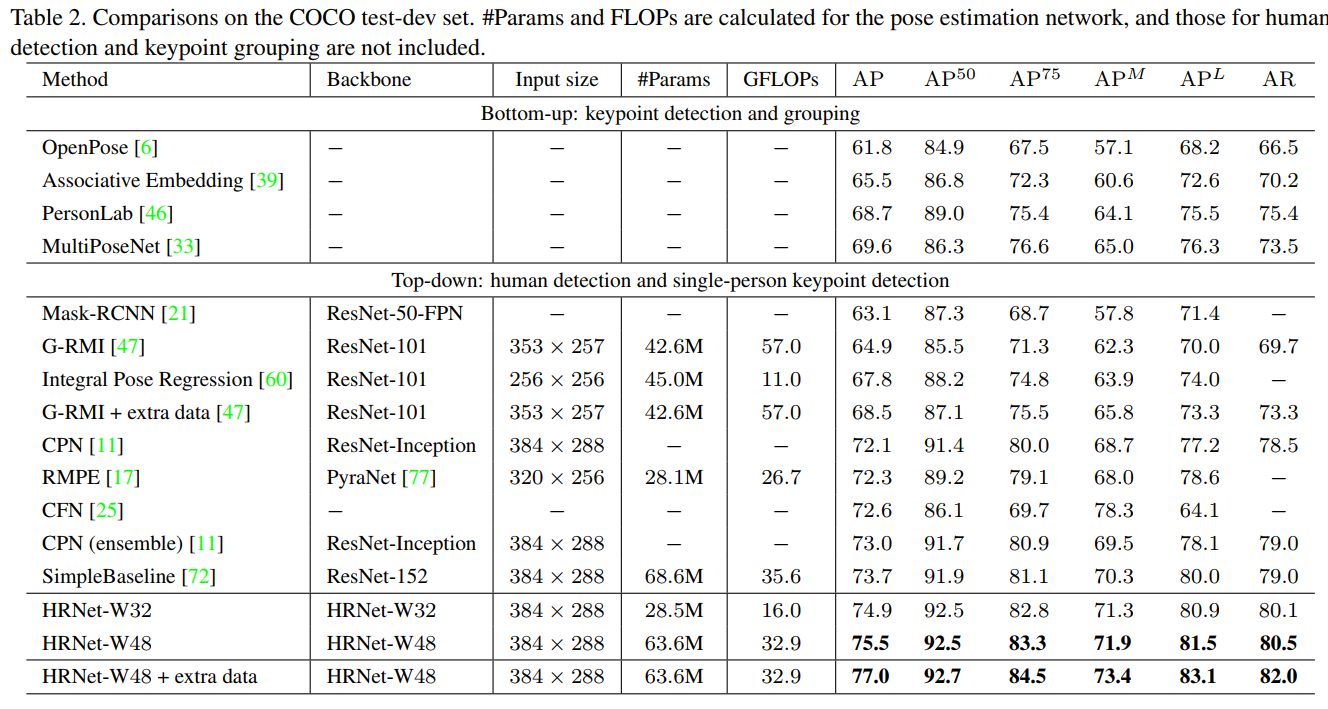
附录:
各种网络的不同分辨率的组合融合方式
High-to-low and low-to-high.
high-to-low process的目标是生成低分辨率和高分辨率的表示,low-to-high process的目标是生成高分辨率的表示[4,11,23,72,40,62]。这两个过程可能会重复多次,以提高性能[77,40,14]。
具有代表性的网络设计模式包括:
(i)Symmetric high-to-low and low-to-high processes。Hourglass及其后续论文[40,14,77,31]将low-to-high proces设计为high-to-low process的镜子。
(ii)Heavy high-to-low and light low-to-high。high-to-low process是基于ImageNet分类网络,如[11,72]中使用的ResNet,low-to-high process是简单的几个双线性上采样[11]或转置卷积[72]层。
(iii)Combination with dilated convolutions。在[27,51,35]中,ResNet或VGGNet在最后两个阶段都采用了扩张性卷积来消除空间分辨率的损失,然后采用由light lowto-high process来进一步提高分辨率,避免了仅使用dilated convolutions的昂贵的计算成本[11,27,51]。图2描述了四种具有代表性的姿态估计网络。
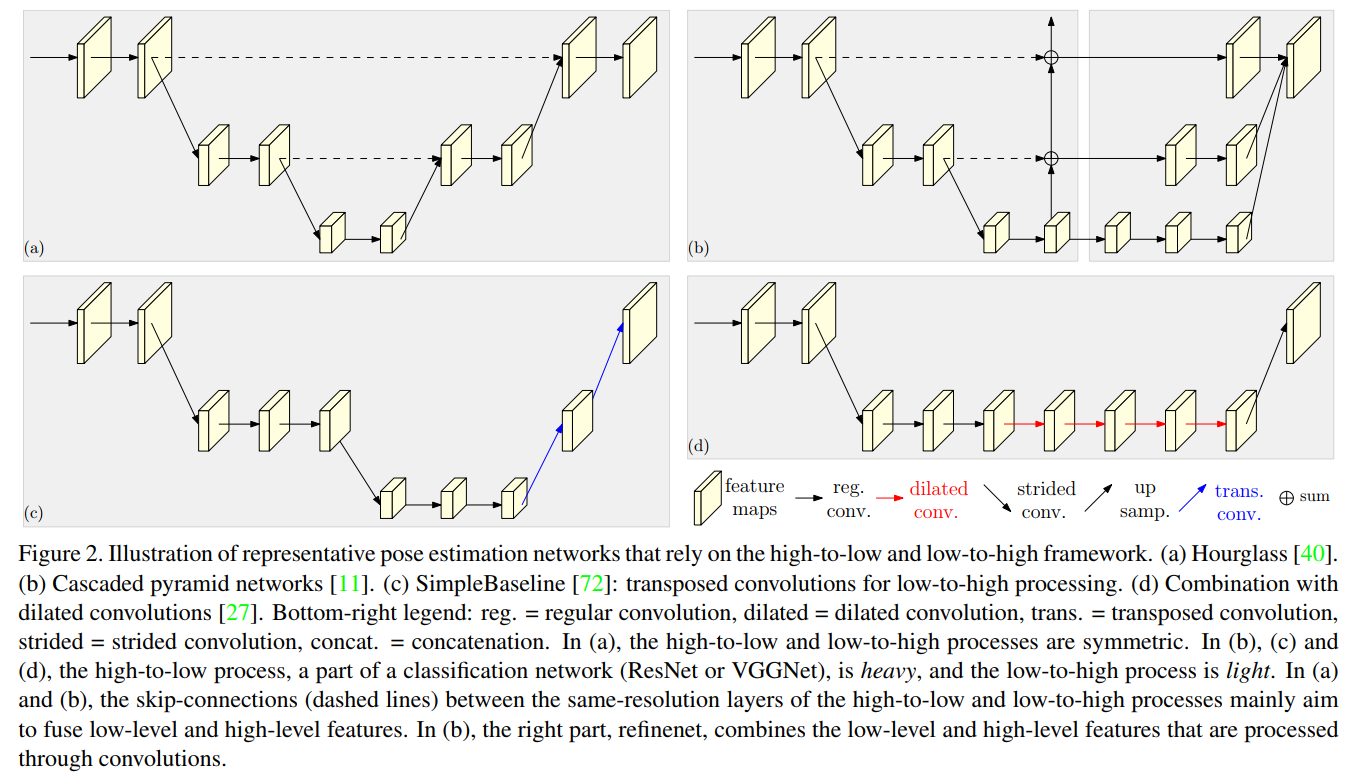
Multi-scale fusion.
最直接的方法是将多分辨率图像分别送入多个网络,并聚合输出响应映射[64]。Hourglass[40]及其扩展[77,31]通过跳过连接,将high-to-low process中的低级别特征逐步组合为low-to-high process中的相同分辨率的高级别特性。在cascaded pyramid network[11]中,globalnet将high-to-low process中的低到高级别特征low-to-high level feature逐步组合到low-to-high process中,refinenet将通过卷积处理的低到高特征进行组合。我们的方法重复多尺度融合,部分灵感来自深度融合及其扩展[67,73,59,80,82]。
参考:
hrnet的pytorch实现:https://github.com/leoxiaobin/deep-high-resolution-net.pytorch/blob/master/lib/models/pose_hrnet.py