机器学习以人为中心的产品交互设计原则
Josh Lovejoy and Jess Holbrook
Machine learning (ML) is the science of helping computers discover patterns and relationships in data instead of being manually programmed. It’s a powerful tool for creating personalized and dynamic experiences, and it’s already driving everything from Netflix recommendations to autonomous cars. But as more and more experiences are built with ML, it’s clear that UXers still have a lot to learn about how to make users feel in control of the technology, and not the other way round.
机器学习是帮助计算机揭示数据模式与关系的科学。机器学习是一个强大的工具,可用于打造个性化的动态体验。机器学习已经有力地推动了从 Netflix 建议到自动驾驶汽车等各项技术的发展。随着使用机器学习打造的产品越来越多,产品的用户体验设计师则需要更多地了解如何让用户感觉自己能轻松驾驭科技。
As was the case with the mobile revolution, and the web before that, ML will cause us to rethink, restructure, displace, and consider new possibilities for virtually every experience we build. In the Google UX community, we’ve started an effort called “human-centered machine learning” (HCML) to help focus and guide that conversation. Using this lens, we look across products to see how ML can stay grounded in human needs while solving them in unique ways only possible through ML. Our team at Google works with UXers across the company to bring them up to speed on core ML concepts, understand how to integrate ML into the UX utility belt, and ensure ML and AI are built in inclusive ways.
正如移动革命以及之前网络技术的兴起一样,机器学习将促使我们对我们构建的几乎所有体验进行反思、重构、置换和考量,从而开辟全新可能。在 Google 用户体验社区,我们启动了一个“以人为中心的机器学习”(HCML - Human-centered machine learning) 项目,旨在帮助聚焦和引导上述对话。从这个角度出发,我们审视了各个产品,以查看机器学习如何能够以人类需求为中心并以机器学习所独有的方式来满足这些需求。在 Google,我们团队与公司内部用户体验设计者合作,探讨如何加速核心机器学习概念的开发,了解如何将机器学习集成到用户体验工具腰带中以及如何确保以包容的方式构建机器学习和人工智能。
If you’ve just started working with ML, you may be feeling a little overwhelmed by the complexity of the space and the sheer breadth of opportunity for innovation. Slow down, give yourself time to get acclimated, and don’t panic. You don’t need to reinvent yourself in order to be valuable to your team.
如果您刚刚开始接触机器学习,机器学习领域的复杂性以及无限的创新机遇可能让您感觉无所适从。不必恐慌,先停下来,给自己留点时间适应一下。您并不需要从头开始,也可以在团队中发挥重要作用。
We’ve developed seven points to help designers navigate the new terrain of designing ML-driven products. Born out of our work with UX and AI teams at Google (and a healthy dose of trial and error), these points will help you put the user first, iterate quickly, and understand the unique opportunities ML creates.
为了帮助设计师适应设计机器学习驱动的产品这一全新领域,我们提出了七个观点。这些观点源自我们与 Google 用户体验和人工智能团队的合作(并进行了必要的试验,允许合理的错误数量),将帮助您遵循用户优先的原则,实现快速迭代,了解机器学习带来的独特机遇。
Let’s get started.
我们开始吧。
1. Don’t expect Machine learning to figure out what problems to solve
1. 不要指望机器学习去思考它要解决什么问题
Machine learning and artificial intelligence have a lot of hype around them right now. Many companies and product teams are jumping right into product strategies that start with ML as a solution and skip over focusing on a meaningful problem to solve.
现在,围绕机器学习和人工智能的宣传铺天盖地,天花乱坠。许多公司和产品团队不是首先关注他们要解决什么重大问题,而是一开始就直奔以机器学习作为解决方案的产品战略。
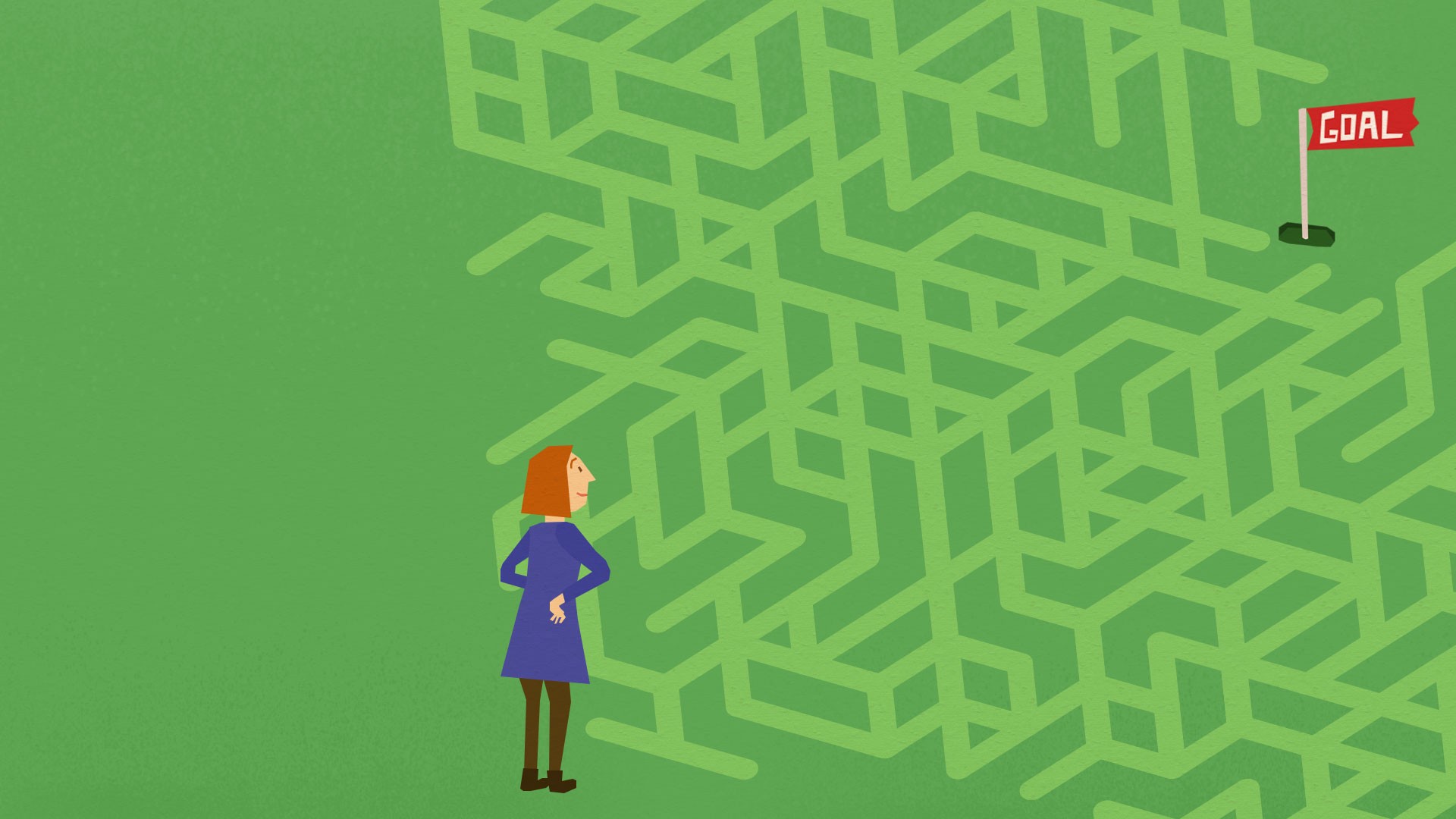
That’s fine for pure exploration or seeing what a technology can do, and often inspires new product thinking. However, if you aren’t aligned with a human need, you’re just going to build a very powerful system to address a very small — or perhaps nonexistent — problem.
这种模式对纯粹探索或了解某项技术的作用很有用,并且常常会启迪对新产品的思索。然而,如果您并未瞄准人类需求,那么您只是在构建一套非常强大的系统来解决一个很小、也许根本不存在的问题。
So our first point is that you still need to do all that hard work you’ve always done to find human needs. This is all the ethnography, contextual inquiries, interviews, deep hanging out, surveys, reading customer support tickets, logs analysis, and getting proximate to people to figure out if you’re solving a problem or addressing an unstated need people have. Machine learning won’t figure out what problems to solve. We still need to define that. As UXers, we already have the tools to guide our teams, regardless of the dominant
technology paradigm.
因此,我们的第一个观点便是您仍然需要一如既往地努力发掘人类的需求。这涉及到人种学、背景查询、采访、深入研究、调研、查阅客户支持服务单据、日志分析并与人们接触,以弄清楚您是否正在解决某个问题或是满足某个未曾言明的人类需求。不要指望机器学习去思考它要解决什么问题。我们仍然需要定义问题。作为用户体验设计者,我们已经拥有指导我们团队的工具,无论主流的技术范式为何。
2. Ask yourself if ML will address the problem in a unique way
2. 询问自己机器学习是否能以独特方式解决问题
Once you’ve identified the need or needs you want to address, you’ll want to assess whether ML can solve these needs in unique ways. There are plenty of legitimate problems that don’t require ML solutions.
一旦您确认您需要满足哪些需求之后,您将需要评估机器学习是否能够以独特方式满足这些需求。有许多符合前述条件的问题并不需要机器学习解决方案。
A challenge at this point in product development is determining which experiences require ML, which are meaningfully enhanced by ML, and which do not benefit from ML or are even degraded by it. Plenty of products can feel “smart” or “personal” without ML. Don’t get pulled into thinking those are only possible with ML.
目前,产品开发中的一大挑战是确定哪些体验需要机器学习,哪些体验可通过机器学习得到实质性增强,哪些体验不能通过机器学习得到改善,甚至会反受其害。有很多产品无需使用机器学习,也可以让人感觉很“智能”或“人性化”。不要想当然地认为只有机器学习才能解决问题。
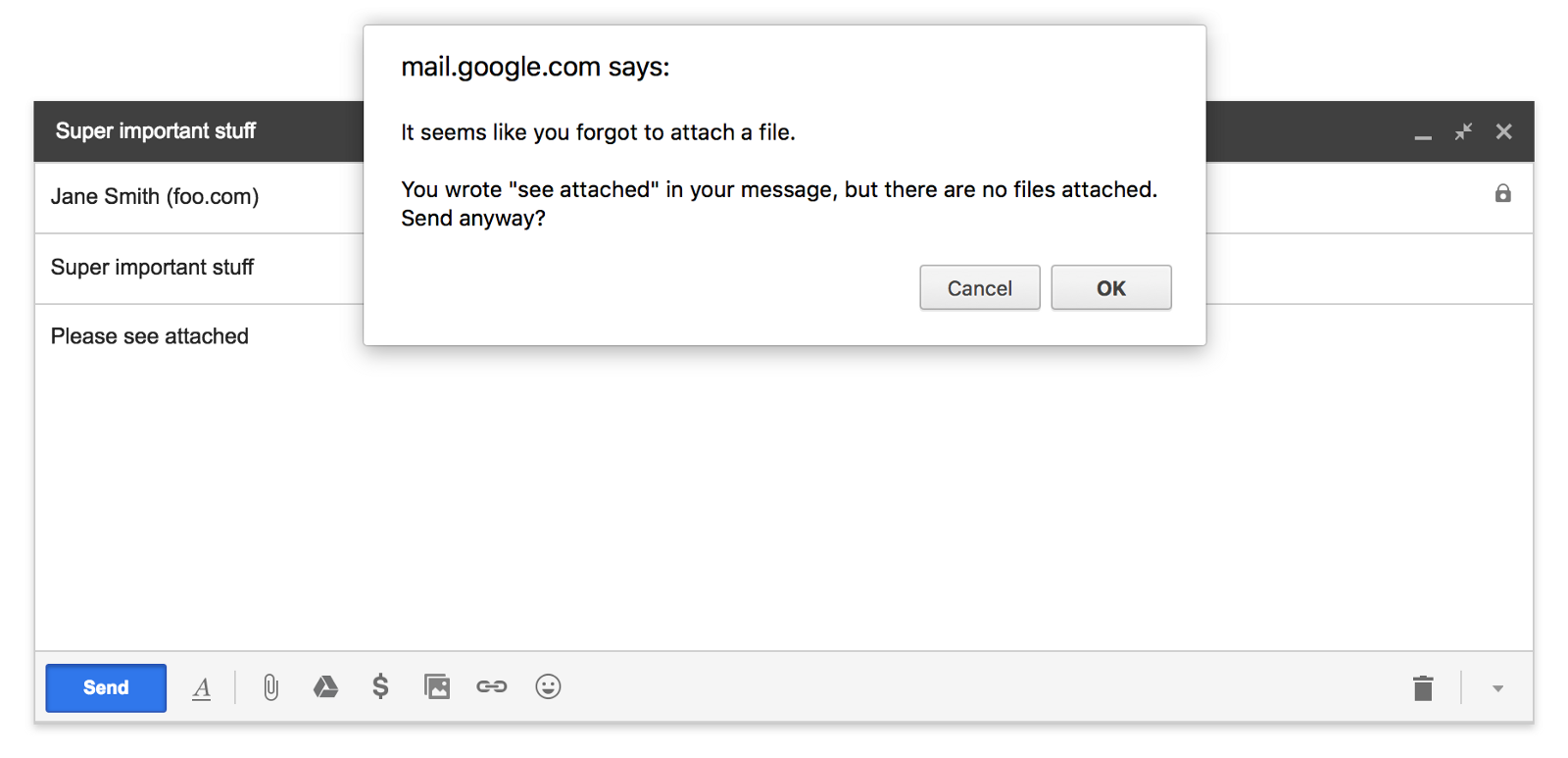
We’ve created a set of exercises to help teams understand the value of ML to their use cases. These exercises do so by digging into the details of what mental models and expectations people might bring when interacting with an ML system as well as what data would be needed for that system.
我们设计了一组练习来帮助团队理解机器学习对于其用例的价值。为此,这些练习深入探究人们在与机器学习系统交互时可能提出的具体心智模式和期望以及该系统需要哪些数据。
Here are three example exercises we have teams walk through and answer about the use cases they are trying to address with ML:
以下是我们让团队围绕他们要使用机器学习解决的用例进行研究和解答的三个示例练习:
Describe the way a theoretical human “expert” might perform the task today.
描述理论上的人类“专家”目前执行此任务的方法。
If your human expert were to perform this task, how would you respond to them so they improved for the next time? Do this for all four phases of the confusion matrix.
如果您的人类专家要执行此任务,您如何为他们提供响应,以便他们在下一次能改进方法?对于混淆矩阵中的所有四个阶段均执行上述步骤。
If a human were to perform this task, what assumptions would the user want them to make?
如果由人类来执行此项任务,用户会希望他们做出哪些假设?
Spending just a few minutes answering each of these questions reveals the automatic assumptions people will bring to an ML-powered product. They are equally good as prompts for a product team discussion or as stimuli in user research. We’ll also touch on these a bit later when we get into the process of defining labels and training models.
请花几分钟时间回答各个问题,了解人类能为机器学习驱动的产品提供的自动假设。这些假设如同产品团队在探讨产品时的提示一样重要,或者如同用户研究时的刺激一样有用。我们稍后讲解定义标签和训练模型时还会再讲到这方面的内容。
After these exercises and some additional sketching and storyboarding of specific products and features, we then plot out all of the team’s product ideas in a handy 2x2:
在完成这些练习以及特定产品和功能的其他一些草图和串连图板后,我们将团队关于产品的各种想法分别写入一个简单的2x2 网格中:
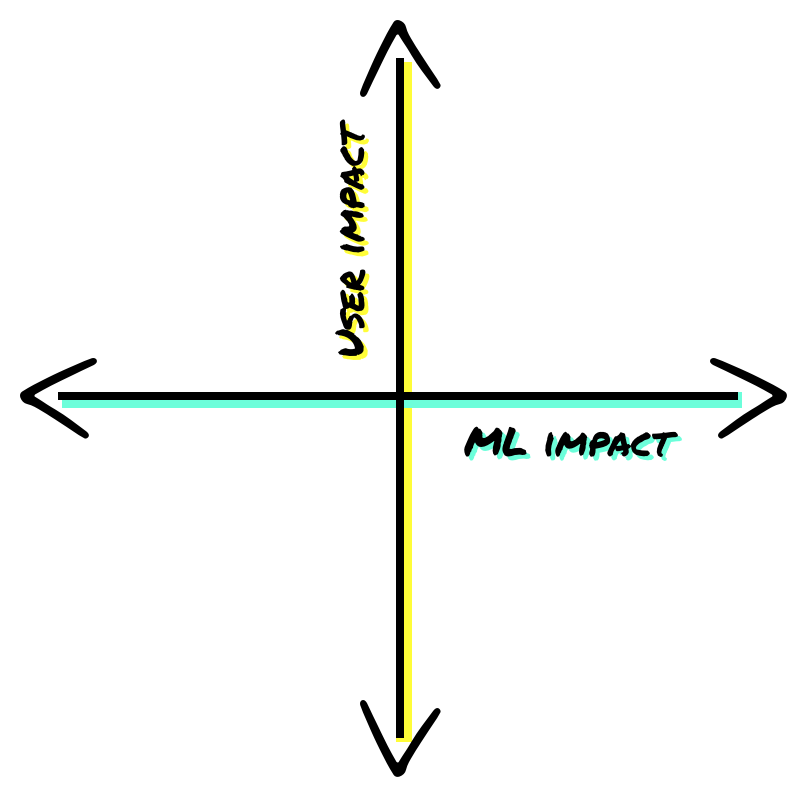
将想法写入这个 2x2 网格中。让团队投票选择哪些想法能够给用户带来最大的影响,哪些想法通过机器学习解决方案可以得到最大程度的加强。
This allows us to separate impactful ideas from less impactful ones as well as see which ideas depend on ML vs. those that don’t or might only benefit slightly from it. You should already be partnering with Engineering in these conversations, but if you aren’t, this is a great time to pull them in to weigh-in on the ML realities of these ideas. Whatever has the greatest user impact and is uniquely enabled by ML (in the top right corner of the above matrix) is what you’ll want to focus on first.
这有助于我们区分作用显著的想法和作用不大的想法,区分能够通过机器学习加以增强的想法和机器学习对其无甚帮助或帮助不大的想法。在这些对话期间,您应该已经开始与工程团队合作,但如果您尚未开始合作,那现在正是参照机器学习的发展现状衡量一下这些想法的好时机。您应当首先专注于机器学习能够以独特方式给用户带来最大影响(见上述矩阵右上角)的想法。
3. Fake it with personal examples and wizards
3. 利用个人数据和向导进行模仿
A big challenge with ML systems is prototyping. If the whole value of your product is that it uses unique user data to tailor an experience to her, you can’t just prototype that up real quick and have it feel anywhere near authentic. Also, if you wait to have a fully built ML system in place to test the design, it will likely be too late to change it in any meaningful way after testing. However, there are two user research approaches that can help: using personal examples from participants and Wizard of Oz studies.
原型设计是机器学习系统面临的一大挑战。如果您的产品的所有价值在于使用独特的用户数据来打造专属定制体验,那就注定不能快速设计出贴近真实的原型。同样,如果您等待建成一套齐全的机器学习系统来测试设计,那在测试完毕后再进行任何有意义的更改很可能都为时已晚。但有两种用户研究方法可以帮助您:使用参与者的个人案例和 Oz 研究向导。
When doing user research with early mockups, have participants bring in some of their own data — e.g. personal photos, their own contact lists, music or movie recommendations they’ve received — to the sessions. Remember, you’ll need to make sure you fully inform participants about how this data will be used during testing and when it will be deleted. This can even be a kind of fun “homework” for participants before the session (people like to talk about their favorite movies after all).
在利用早期模型进行用户研究时,让参与者为课程提供一些他们自己的数据,例如个人照片、联系人列表,或者他们收到的音乐或电影建议。请记住,务必明确完整地告知参与者在测试期间会如何使用这些数据以及何时删除这些数据。您甚至可以将这作为参与者的趣味“家庭作业”,要求他们在课前完成(毕竟大家都喜欢讨论自己喜欢的电影)。
With these examples, you can then simulate right and wrong responses from the system. For example, you can simulate the system returning the wrong movie recommendation to the user to see how she reacts and what assumptions she makes about why the system returned that result. This helps you assess the cost and benefits of these possibilities with much more validity than using dummy examples or conceptual descriptions.
您可以利用这些示例数据来模拟系统的正确或错误响应。例如,您可以模拟系统向用户推荐不合适的电影来观察其反应,了解用户对系统返回该结果的原因做出的假设。相比使用虚拟数据或概念性说明,这种方法能够帮助您更有效地评估这些可能性涉及的成本与收益。
The second approach that works quite well for testing not-yet-built ML products is conducting Wizard of Oz studies. All the rage at one time, Wizard of Oz studies fell from prominence as a user research method over the past 20 years or so. Well, they’re back.
第二种有效测试尚未成型的机器学习产品的方法是执行 Oz 研究向导。这种用户研究方法曾经十分流行,但在过去约 20 年间渐失风头。现在它们又卷土重来了。
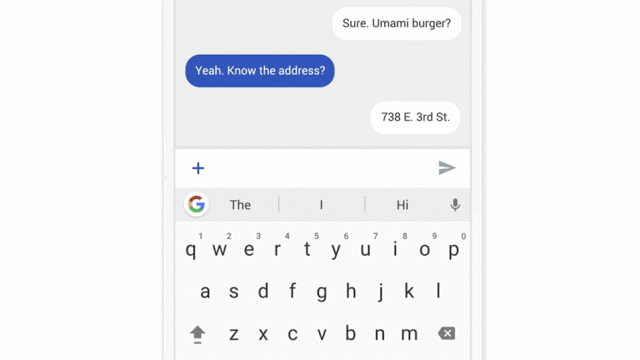
适合使用 Oz 向导方法测试的最简单的体验之一便是聊天界面。只需安排团队成员在聊天的另一端输入“人工智能”提供的“回答”。
Quick reminder: Wizard of Oz studies have participants interact with what they believe to be an autonomous system, but which is actually being controlled by a human (usually a teammate).
快速提醒:Oz 研究向导参与者认为自己是在与自主系统进行互动,但系统其实是由人控制的(通常由团队成员控制)。
Having a teammate imitate an ML system’s actions like chat responses, suggesting people the participant should call, or movies suggestions can simulate interacting with an “intelligent” system. These interactions are essential to guiding the design because when participants can earnestly engage with what they perceive to be an AI, they will naturally tend to form a mental model of the system and adjust their behavior according to those models. Observing their adaptations and second-order interactions with the system are hugely valuable to informing its design.
让团队成员模仿机器学习系统的操作(例如聊天回复,建议参与者应该给谁拨打电话,或者电影建议),可以模拟与“智能”系统互动。这些互动对于指导设计思路至关重要,因为参与者真正认为自己是在与人工智能互动时,他们会自然而然地形成系统的心智模式,并根据这些模式调整自己的行为。观察他们的调整以及与系统的二阶互动非常有助于启迪设计灵感。
4. Weigh the costs of false positives and false negatives
4. 权衡误报和漏报的成本
Your ML system will make mistakes. It’s important to understand what these errors look like and how they might affect the user’s experience of the product. In one of the questions in point 2 we mentioned something called the confusion matrix. This is a key concept in ML and describes what it looks like when an ML system gets it right and gets it wrong.
机器学习系统出错在所难免。了解这些错误的性质以及它们对产品用户体验的影响非常重要。我们在第 2 点的一个问题中提到了混淆矩阵这一概念。这是机器学习中的一个关键概念,描述了当机器学习系统判断正确或出错时的情况。
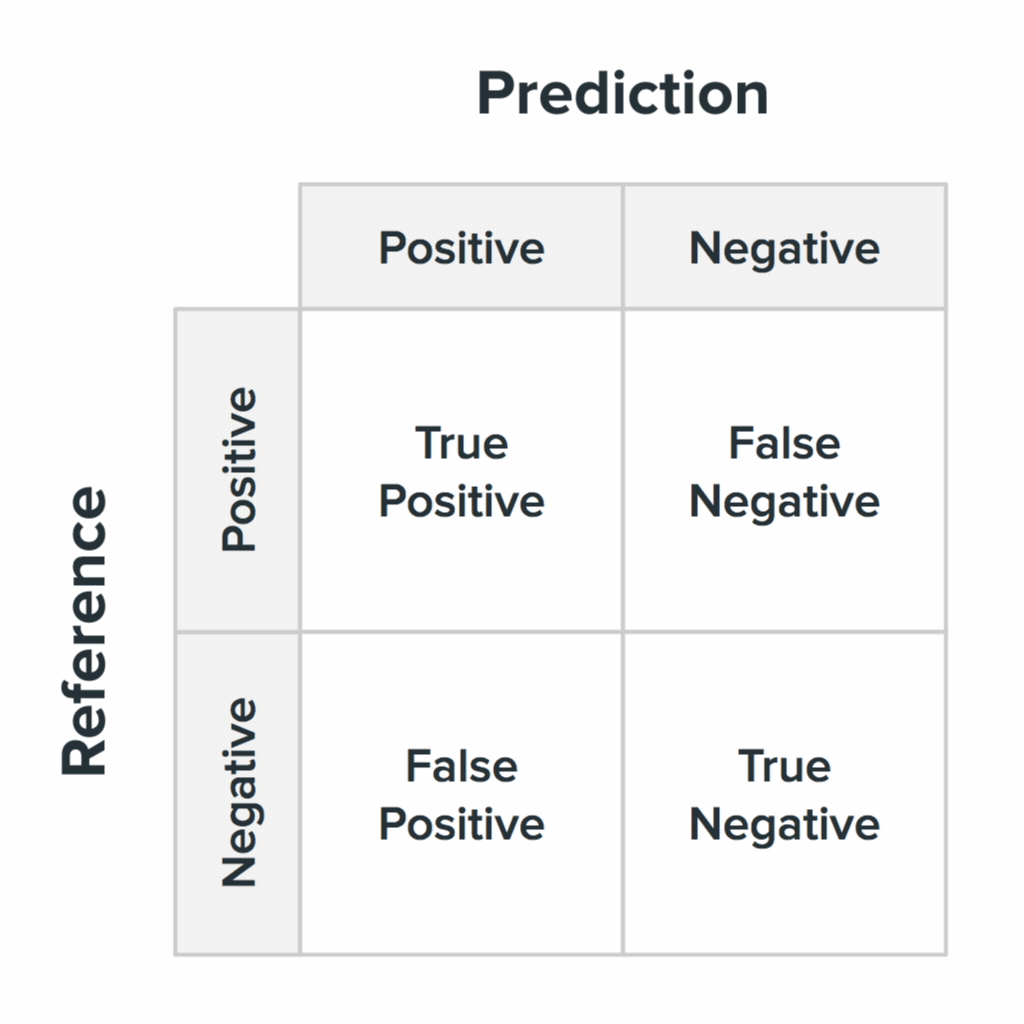
混淆矩阵的四个状态,以及可能对用户意味着什么。
While all errors are equal to an ML system, not all errors are equal to all people. For example, if we had a “is this a human or a troll?” classifier, then accidentally classifying a human as a troll is just an error to the system. It has no notion of insulting a user or the cultural context surrounding the classifications it is making. It doesn’t understand that people using the system may be much more offended being accidentally labeled a troll compared to trolls accidentally being labeled as people. But maybe that’s our people-centric bias coming out. :)
所有错误对于机器学习系统而言没什么不同,而对于人则不是这样。例如,如果有一个“这是人类还是怪物?”的分类器,那么偶然地将一个人归类为怪物对系统来说只是一个错误。它不会认为所做的分类会冒犯用户或其文化背景。它也不会明白,与将怪物意外地标记为人相比,系统用户对于被意外标记为怪物会感到更不舒服。但这可能就是我们以人为中心的偏见。:)
In ML terms, you’ll need to make conscious trade-offs between the precision and recall of the system. That is, you need to decide if it is more important to include all of the right answers even if it means letting in more wrong ones (optimizing for recall), or minimizing the number of wrong answers at the cost of leaving out some of the right ones (optimizing for precision). For example, if you are searching Google Photos for “playground”, you might see results like this:
用机器学习术语来说,您需要有意识地在系统的准确率和召回率之间进行权衡。也就是说,您需要决定是包括所有正确答案重要,即使这意味着会加入较多错误答案(优化召回率),还是宁可遗漏一些正确答案也要最大程度减少错误答案(优化准确率)重要。例如,如果您在 Google 照片中搜索“游乐场”,您可能会看到如下结果:
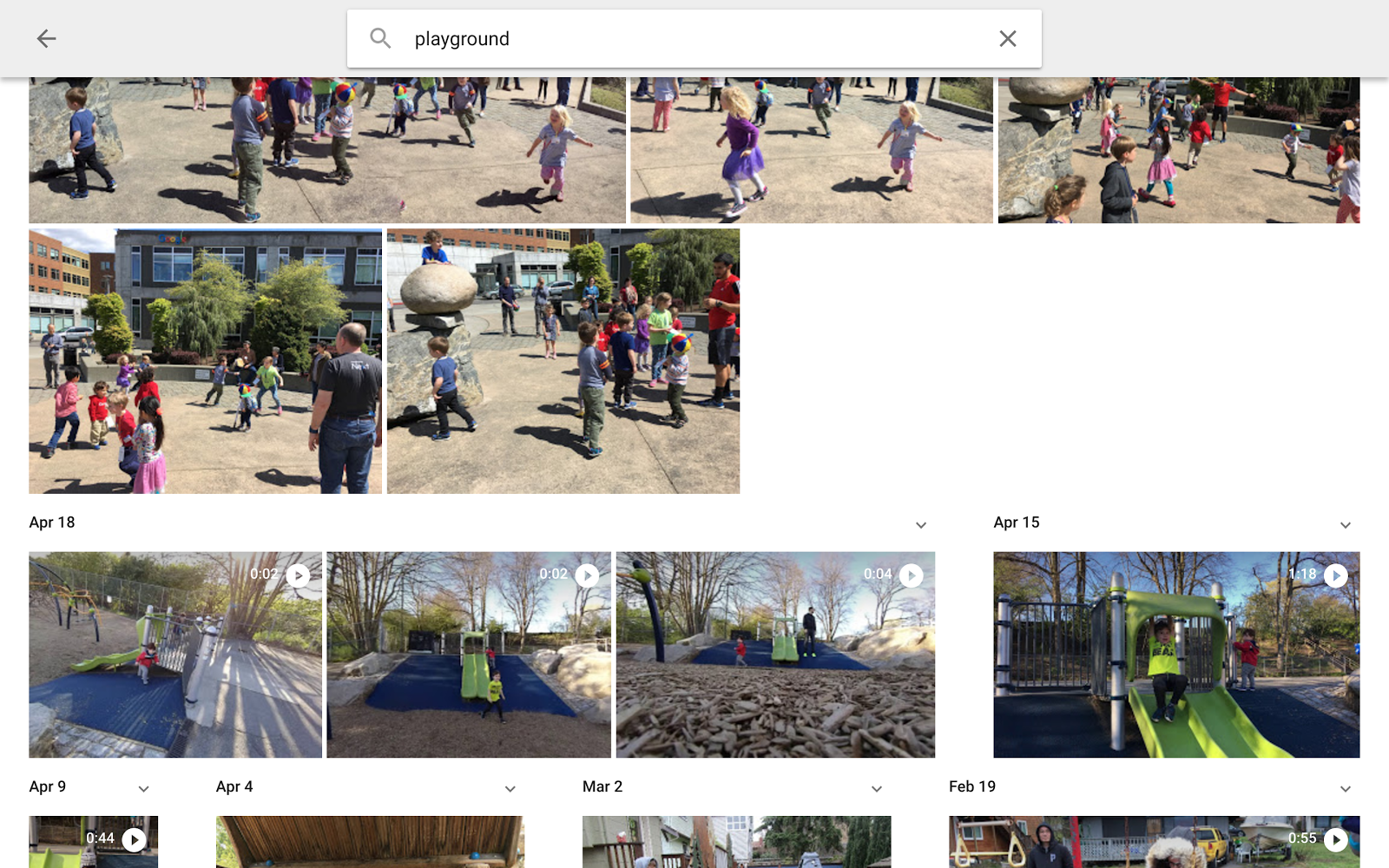
These results include a few scenes of children playing, but not on a playground. In this case, recall is taking priority over precision. It is more important to get all of the playground photos and include a few that are similar but not exactly right than it is to only include playground photos and potentially exclude the photo you were looking for.
这些结果包含几个儿童玩耍的场景,但不是在游乐场上。在此案例中,召回率优先于准确率。与仅包含游乐场照片但可能排除了您要寻找的照片相比,获取所有游乐场照片但包含几个相似但不完全正确的照片更重要。
5. Plan for co-learning and adaptation
5. 规划共同学习和调整
The most valuable ML systems evolve over time in tandem with users’ mental models. When people interact with these systems, they’re influencing and adjusting the kinds of outputs they’ll see in the future. Those adjustments in turn will change how users interact with the system, which will change the models… and so on, in a feedback loop. This can result in “conspiracy theories” where people form incorrect or incomplete mental models of a system and run into problems trying to manipulate the outputs according to these imaginary rules. You want to guide users with clear mental models that encourage them to give feedback that is mutually beneficial to them and the model.
最有价值的机器学习系统应随着时间的推移与用户的心智模式交替进化。当人们与这些系统进行交互时,他们也在影响和调整着他们将来看到的各种系统输出。这些调整反过来将改变用户与系统的交互方式,从而改变心智模式,如此反复,形成一个反馈环路。这可能会导致“阴谋论”,人们使系统形成不正确或不完整的心智模式,并会遇到试图根据这些虚构的规则操控输出的问题。您需要使用明确的心智模式引导用户,鼓励他们提供有利于他们自己和模式的反馈。
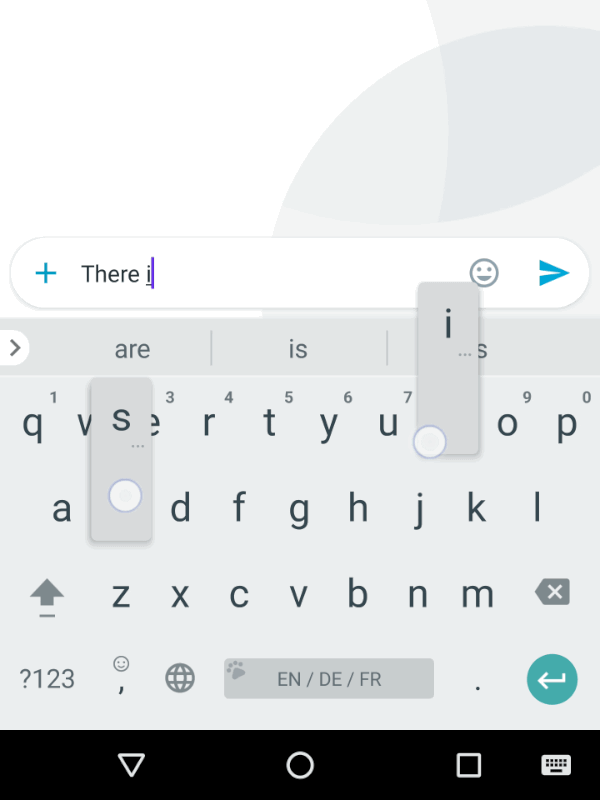
这种良性循环的一个例子是 Gboard 能不断进化,以预测用户要输入的下一个字词。用户使用系统建议的次数越多,系统给出的建议就越好。
While ML systems are trained on existing data sets, they will adapt with new inputs in ways we often can’t predict before they happen. So we need to adapt our user research and feedback strategies accordingly. This means planning ahead in the product cycle for longitudinal, high-touch, as well as broad-reach research together. You’ll need to plan enough time to evaluate the performance of ML systems through quantitative measures of accuracy and errors as users and use cases increase, as well as sit with people while they use these systems to understand how mental models evolve with every success and failure.
机器学习系统是基于现有数据集训练的,因此,我们通常无法预测系统将如何针对新输入进行调整。因此,我们需要相应调整我们的用户研究和反馈策略。这意味着我们需要在产品周期中提前规划开展纵向的、人机交互频繁的广泛研究。随着用户和用例不断增多,您需要预留充足的时间,通过定量分析准确率和错误率来评估机器学习系统的性能,并在用户使用这些系统时与他们坐在一起,以了解他们的心智模式如何随着每一次成功或失败而发生变化。
Additionally, as UXers we need to think about how we can get in situ feedback from users over the entire product lifecycle to improve the ML systems. Designing interaction patterns that make giving feedback easy as well as showing the benefits of that feedback quickly, will start to differentiate good ML systems from great ones.
此外,作为用户体验设计者,我们需要考虑如何能够在整个产品生命周期内获得用户的真实反馈,以改进机器学习系统。机器学习系统是良好还是卓越,区别在于设计的交互模式是否方便提供反馈以及是否能够快速显示反馈的好处。
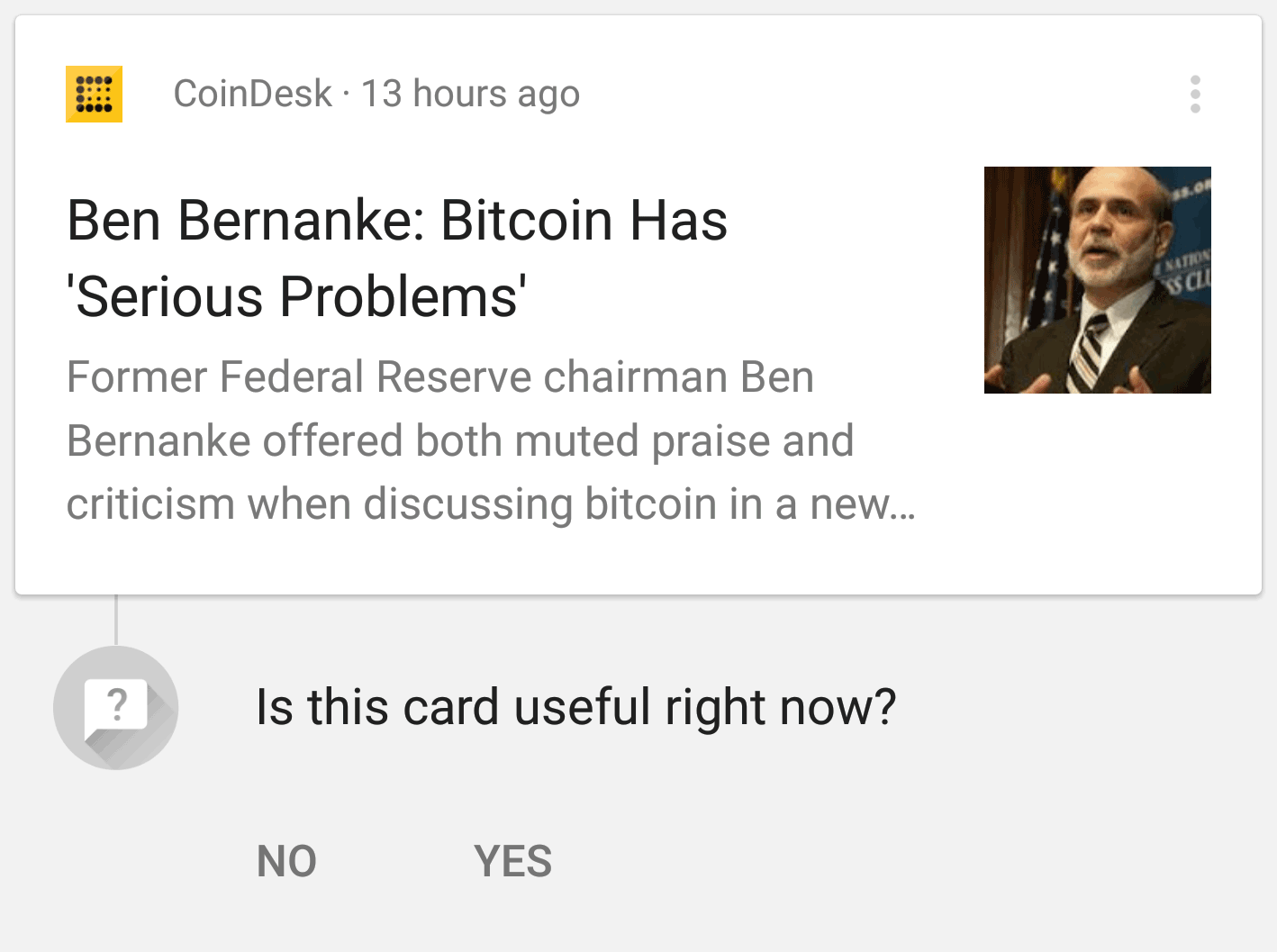
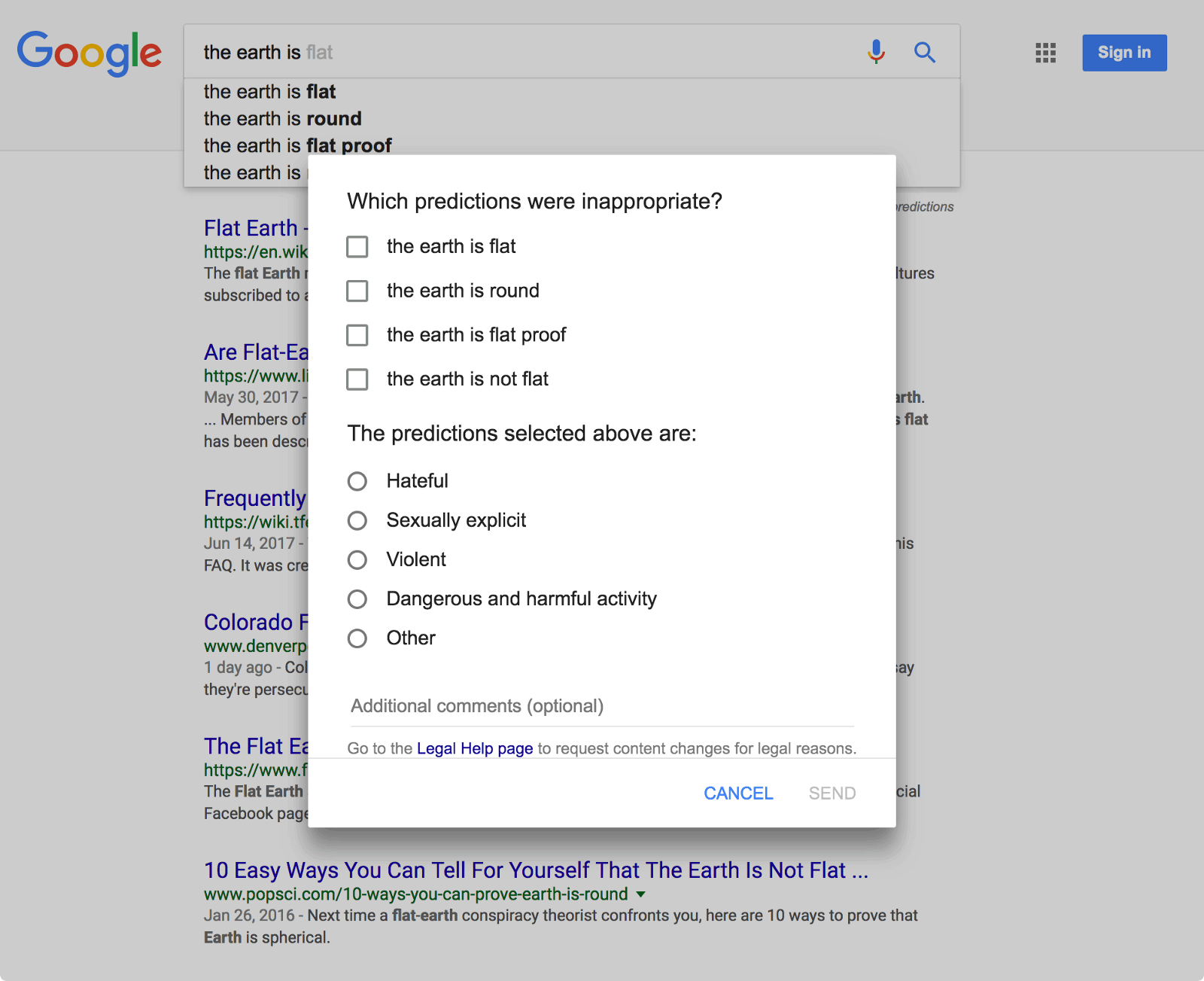
6. Teach your algorithm using the right labels
6. 使用正确的标签训练算法
As UXers, we’ve grown accustomed to wireframes, mockups, prototypes, and redlines being our hallmark deliverables. Well, curveball: when it comes to ML-augmented UX, there’s only so much we can specify. That’s where “labels” come in.
作为用户体验设计者,我们已经习惯于将线框、模型、原型和红线作为我们的标志性交付成果。然而,蹊跷的是:当谈到通过机器学习增强的用户体验时,我们能够侃侃而谈的寥寥无几。这时“标签”就派上用场了。
Labels are an essential aspect of machine learning. There are people whose job is to look at tons of content and label it, answering questions like “is there a cat in this photo?” And once enough photos have been labeled as “cat” or “not cat”, you’ve got a data set you can use to train a model to be able to recognize cats. Or more accurately, to be able to predict with some confidence level whether or not there’s a cat in a photo it’s never seen before. Simple, right?
标签是机器学习不可或缺的一部分。有些人的工作就是查看大量内容并为内容添加标签,回答一些类似“这张照片中是否有一只猫?”的问题。一旦将足够的照片标记为“猫”或“非猫”,您就会获得一个数据集,您可以用它来训练模型识别猫。或者更准确地说,能够让模型以一定的置信水平预测一张它从未见过的照片中是否有猫。很简单,对不对?
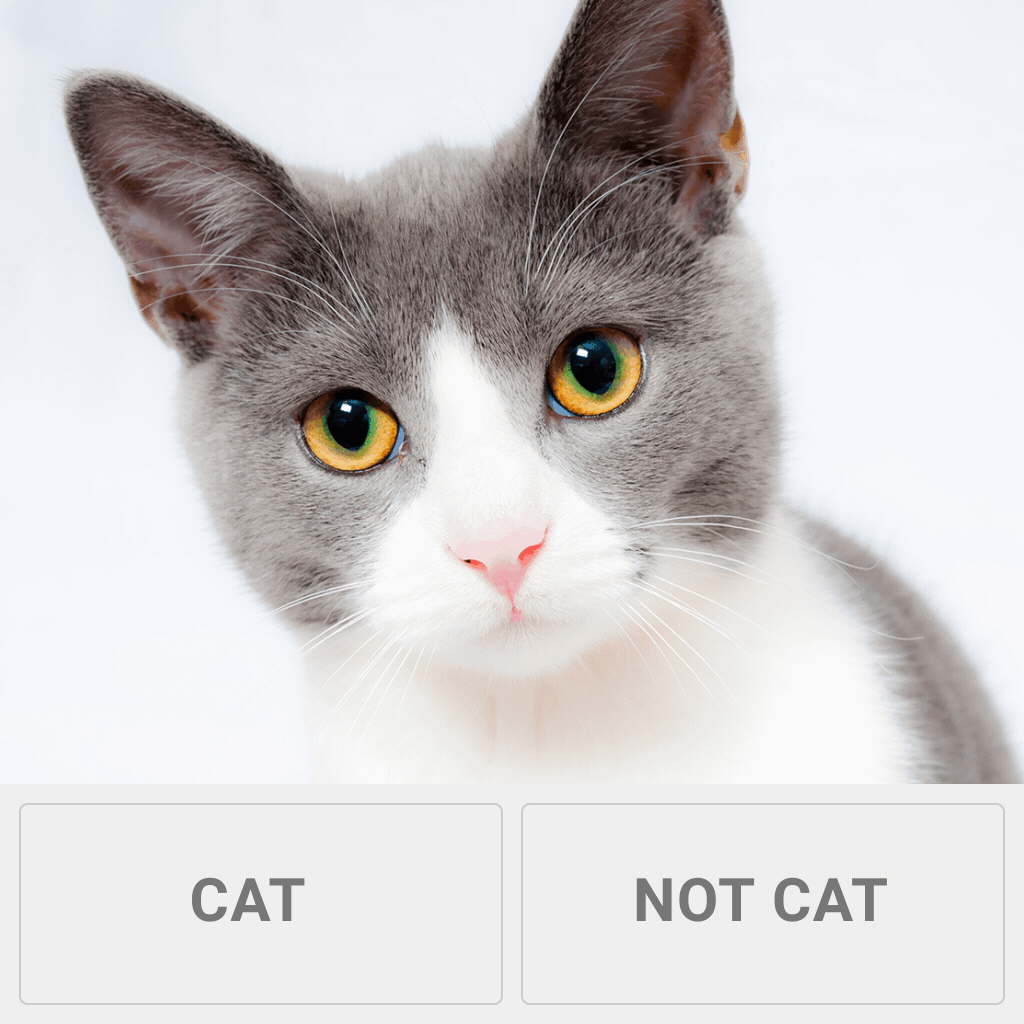
您能通过这个测验吗?
The challenge comes when you venture into territory where the goal of your model is to predict something that might feel subjective to your users, like whether or not they’ll find an article interesting or a suggested email reply meaningful. But models take a long time to train, and getting a data set fully labeled can be prohibitively expensive, not to mention that getting your labels wrong can have a huge impact on your product’s viability.
当您冒险进入模型目标定是预测一些对用户来说很主观的东西(如他们是否认为某篇文章很有趣或建议的电子邮件回复有意义)的领域时,挑战就来了。而且模型需要长时间的训练,获得一个完全标记的数据集的成本可能非常高昂,更不用说标签错误将给您的产品可行性带来巨大影响。
So here’s how to proceed: Start by making reasonable assumptions and discussing those assumptions with a diverse array of collaborators. These assumptions should generally take the form of “for ________ users in ________ situations, we assume they’ll prefer ________ and not ________.” Then get these assumptions into the hackiest prototype possible as quickly as possible in order to start gathering feedback and iterating.
以下是应采取的做法:首先进行合理的假设,然后与各种协作者对这些假设进行讨论。这些假设通常应采用的格式为:“对于处于 _______ 情况中的_______ 用户,我们假设他们更喜欢 _______ 而不是 _______。”然后,尽快将这些假设置于使用最频繁的原型里,以开始收集反馈并进行迭代。
Find experts who can be the best possible teachers for your machine learner — people with domain expertise relevant to whatever predictions you’re trying to make. We recommend that you actually hire a handful of them, or as a fallback, transform someone on your team into the role. We call these folks “Content Specialists” on our team.
聘请可以充当机器学习最佳老师的专家,即具有您尝试预测的相关领域专业知识的人员。我们建议您聘请多名专家,或让您团队中的某人作为后备人员担任该职位。在我们团队,我们称这些人为“内容专家”。
By this point, you’ll have identified which assumptions are feeling “truthier” than others. But before you go big and start investing in large-scale data collection and labeling, you’ll want to perform a critical second round of validation using examples that have been curated from real user data by Content Specialists. Your users should be testing out a high-fidelity prototype and perceive that they’re interacting with a legit AI (per point #3 above).
此时,您将会确定哪些假设看起来更加“真实”。然而,在您开始大规模收集数据和进行标记之前,您需要使用由内容专家从真实的用户数据中挑选的示例进行关键的第二轮验证。用户应测试一个高保真的原型,并知道自己正与一个合法的人工智能交互(根据上面的第 3 点)。
With validation in-hand, have your Content Specialists create a broad portfolio of hand-crafted examples of what you want your AI to produce. These examples give you a roadmap for data collection, a strong set of labels to start training models, and a framework for designing large scale labeling protocols.
通过实操验证,让您的内容专家针对您希望您的人工智能生成的内容创建大量手工设计的示例组合。这些示例将为您提供一个数据收集路线图、一套用于开始训练模型的强大标签集,以及一个用于设计大规模标记协议的框架。
7. Extend your UX family, ML is a creative process
7. 拓展您的用户体验系列,机器学习是一个创造性过程
Think about the worst micro-management “feedback” you’ve ever received as a UXer. Can you picture the person leaning over your shoulder and nit-picking your every move? OK, now keep that image in your mind… and make absolutely certain that you don’t come across like that to your engineers.
考虑您作为一个用户体验设计者所收到的最糟糕的微观管理“反馈”。您能想象一个人靠在您的肩膀上,然后您每动一下就对您吹毛求疵吗?好,现在继续在您的头脑中想象该画面,您一定不想遇到这样的人,您的工程师也是。
There are so many potential ways to approach any ML challenge, so as a UXer, getting too prescriptive too quickly may result in unintentionally anchoring — and thereby diminishing the creativity of — your engineering counterparts. Trust them to use their intuition and encourage them to experiment, even if they might be hesitant to test with users before a full evaluation framework is in place.
有许多潜在的方法都可以应对机器学习挑战,因此,作为一个用户体验设计者,太过规范化或进度太快可能导致过程意外地停顿,从而削弱了您的工程师同事的创造力。相信他们的直觉,并鼓励他们进行实验,即使在完整评估框架到位之前他们可能对通过用户进行测试犹豫不决。
Machine learning is a much more creative and expressive engineering process than we’re generally accustomed to. Training a model can be slow-going, and the tools for visualization aren’t great yet, so engineers end up needing to use their imaginations frequently when tuning an algorithm (there’s even a methodology called “Active Learning” where they manually “tune” the model after every iteration). Your job is to help them make great user-centered choices all along the way.
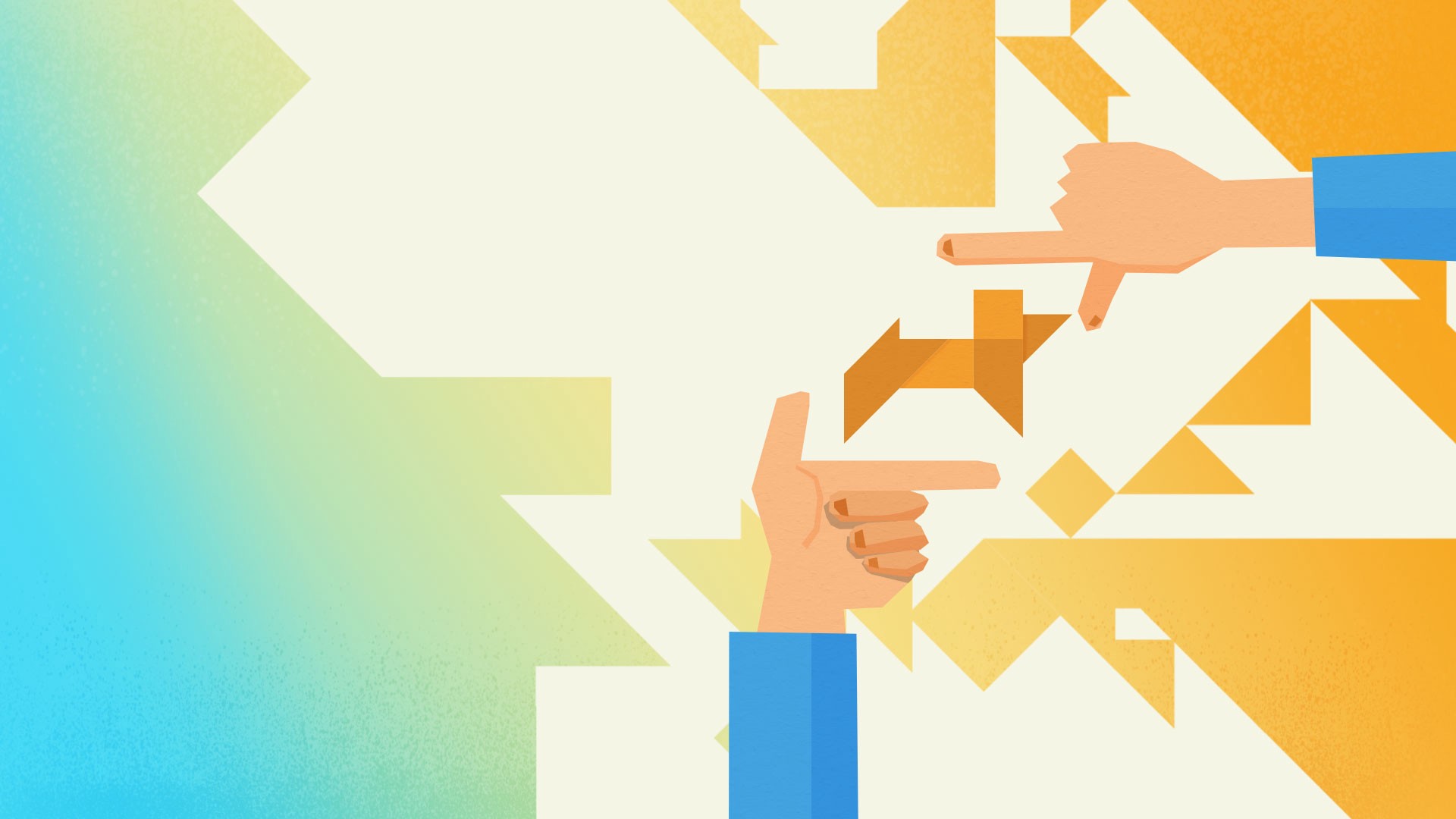
So inspire them with examples — decks, personal stories, vision videos, prototypes, clips from user research, the works — of what an amazing experience could look and feel like, build up their fluency in user research goals and findings, and gently introduce them to our wonderful world of UX crits, workshops, and design sprints to help manifest a deeper understanding of your product principles and experience goals. The earlier they get comfortable with iteration, the better it will be for the robustness of your ML pipeline, and for your ability to effectively influence the product.
因此,可以通过示例—— decks、个人经历、视频、原型、来自用户研究的剪辑、作品等启发工程师,让他们观看和感受什么是出色的体验,熟悉用户研究目标和结果,并礼貌地请他们加入精彩的 UX crits 世界和研讨会,向他们介绍设计冲刺哲学,以帮助他们更深入地理解您的产品原则和体验目标。工程师越早适应迭代,机器学习管道的稳健性就越好,您就越能有效地影响产品。
Conclusion
结论
These are the seven points we emphasize with teams in Google. We hope they are useful to you as you think through your own ML-powered product questions. As ML starts to power more and more products and experiences, let’s step up to our responsibility to stay human-centered, find the unique value for people, and make every experience great.
以上就是我们在 Google 设计产品时与团队强调的七个观点。我们希望它们能帮助您思考您自己基于机器学习的产品。随着机器学习开始支持越来越多的产品和体验,让我们坚守以人为中心的责任,为人们提供独特价值和卓越的体验。
Authors
作者
Josh Lovejoy is a UX Designer in the Research and Machine Intelligence group at Google. He works at the intersection of Interaction Design, Machine Learning, and unconscious bias awareness, leading design and strategy for Google’s ML Fairness efforts.
Josh Lovejoy 是 Google Research 和机器智能小组的一位用户体验设计师。他致力于交互设计、机器学习和无意识偏见觉察的交叉领域的研究,并主导 Google 的机器学习公平性设计和策略工作。
Jess Holbrook is a UX Manager and UX Researcher in the Research and Machine Intelligence group at Google. He and his team work on multiple products powered by AI and machine learning that take a human-centered approach to these technologies.
Jess Holbrook 是 Google Research 和机器智能小组的一位用户体验经理兼用户体验研究员。他和他的团队致力于基于人工智能和机器学习的多种产品的工作,采取以人为中心的方法来处理这些技术。
Akiko Okazaki did the beautiful illustrations.
Akiko Okazaki 制作了精美的插图。
(原文:https://medium.com/google-design/human-centered-machine-learning-a770d10562cd)